The Daft.ie Property Tax Calculator – how does it work?
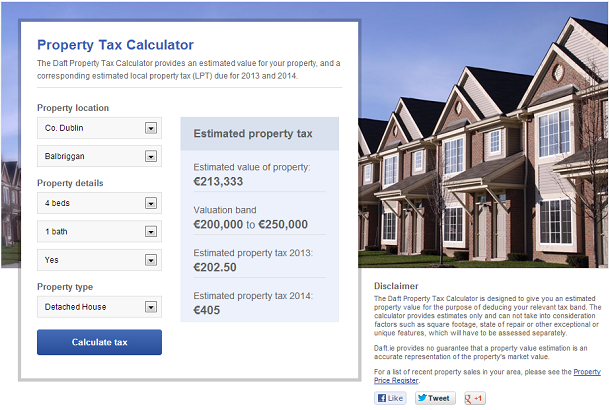
Last week we launched a Property Tax Calculator on Daft.ie. It’s a simple tool which asks for information on a property’s location, bedrooms, bathrooms and type, before giving an estimated value for the property. This in turn calculates the Local Property Tax (LPT) due to be paid when the new scheme starts in July. So, where did the idea come from, and how does it work?
Where did this come from?
One of the conditions of the bailout the Irish government received from the IMF was the introduction of a residential property tax. The tax paid is to be based off a self-assessment of the property’s value. This tax is due to be levied in July this year for the last 6 months of 2013, then from January 2014 be billed annually to cover the full 12 months of the year.
In early March, the Revenue Commissioners released an online tool which aims to help people estimate the value of their property. The Revenue have been at pains to stress that this tool is not definitive, and should be taken only as a starting point for valuation. There was much debate about the accuracy of this tool, due to the limited amount of data it has for each property (area and rough property age). This leads to average prices being shown for an area which may have, for example, both 1 bed apartments and 4 bed semi-detached houses. The value of each of these properties would be wildly different, but the revenue tool shows them both in the same tax band. Not ideal, and it’s caused a lot of confusion for people worried about declaring themselves in the wrong band. The Revenue have publicly acknowledged that up to 50% of properties may be valued in the wrong tax band initially. Potentially a fairly big margin of error there.
One of the big issues the Revenue have is that they just don’t have the data quality needed to carry out this sort of exercise. They may know that a property exists in a given location, but don’t have access to it’s size, type, whether it’s been extended, etc, so they’re starting at a disadvantage here. All of this information exists somewhere within various government departments, but a lack of joined-up data is a recurring theme in Irish government. That’s a rant for another day!
Is there another way to do it?
So, if the Revenue don’t have great data, what can be done? On Daft.ie, we regularly release property market analysis via the Daft Report. These reports are based on the details users enter when placing or editing an ad, so is much richer than the revenue data. We have access to things like bedroom number and property type, which can have a big impact on a property’s value. Daft’s economist Ronan Lyons got to work on a model similar to that behind the Daft Report which would allow us to use the rich data we already have from property listings to hopefully give a more accurate valuation tool.
Ronan split the country in to 5 large regions (Dublin, Other Cities, Leinster, Munster and Connaught-Ulster), as well as in to 365 smaller areas (micro-markets), which are generated by taking the areas offered in the Daft search and grouping them by proximity and similarity. Each of these micro-markets was given a coefficient so that we could say that all else being equal, a property in micro-market A is worth 20% more than micro-market B.
Coefficients were then generated for property features (type, number of beds, baths, presence of a garden) based on the property’s location. Once the hard work of evaluating all of these coefficients is done, they get multiplied together to work out a property’s price relative to a control, base property, and this give’s the property’s approximate current value. One of the drawbacks of using Daft data is that people list the asking prices they hope to achieve on the site, which isn’t always the same as a sale price. Based on 2012 analysis, a 10% discount is applied to allow for the drop between asking and realised prices.
Ronan goes in to a good bit more detail on the ins and outs of how these coefficients were generated in a great post on his site, the summary of it being:
The estimated value of a property is based on an economic hedonic pricing model of all 150,000 listings on the daft.ie site in 2012 and 2013, which controls for when a property was listed, its location, type and size, and other measurable characteristics.
Does it work?
Initial feedback on the calculator has been very positive, through emails to our support team, feedback on social media and nice write-ups from users, such as this one from FeckTV.
We’re finding the Daft property tax calculator more accurate than Revenue – what are your thoughts? bit.ly/Z0QA66
— House and Home (@houseandhomemag) March 13, 2013
DAFT Property Tax Calculator takes number of bedrooms into account, liable to be bit more accurate than Revenue’s daft.ie/property-tax
— Gary in D3 (@garyInD3) March 13, 2013
For those of you having a conniption over the Property Tax, like me, this calculator is a bit more user friendly daft.ie/property-tax?c…
— Nollaig (@nollaigbrennan) March 13, 2013
The Daft.ie Property Tax calculator is way better then the MyHome.ie one.. both way better then the Revenue one! bit.ly/10N8Tfs
— Leon Quinn (@reverbstudios) March 14, 2013
Daft.ie launches its own property tax calculator. It’s much better. ow.ly/iReLi #PropertyTax @daftmedia
— FeckTV.com (@FeckTv) March 13, 2013
There was also a lengthy discussion on the Joe Duffy radio show (Ireland’s most popular phone-in radio show) about the accuracy level of the Daft estimations versus those of the revenue. As an aside, I took a quick look at the traffic impact Joe Duffy has when mentioning a site.
The model is still not perfect – it does not allow for things like the size of the property or improvements made such as a conservatory or good insulation (high energy rating). What we hope that it does do is provide a little more useful information for home-owners ahead of the filing deadline at the end of May.
CyberWiseCon 2025
In May 2025, I'll be presenting Digital Cat-and-Mouse: Strategies to Outsmart Scrapers, Phishers, and Thieves at CyberWiseCon in Vilnius, Lithuania. From selling 10 Downing St, to moving the Eiffel Tower to Dublin, this talk covers real-world examples of unconventional ways to stop scrapers, phishers, and content thieves. You'll gain practical insights to protect assets, outsmart bad actors, and avoid the mistakes we made along the way!
Get your ticket now and I'll see you there!